2024-04-04
AI can make it possible to map all conceivable molecules
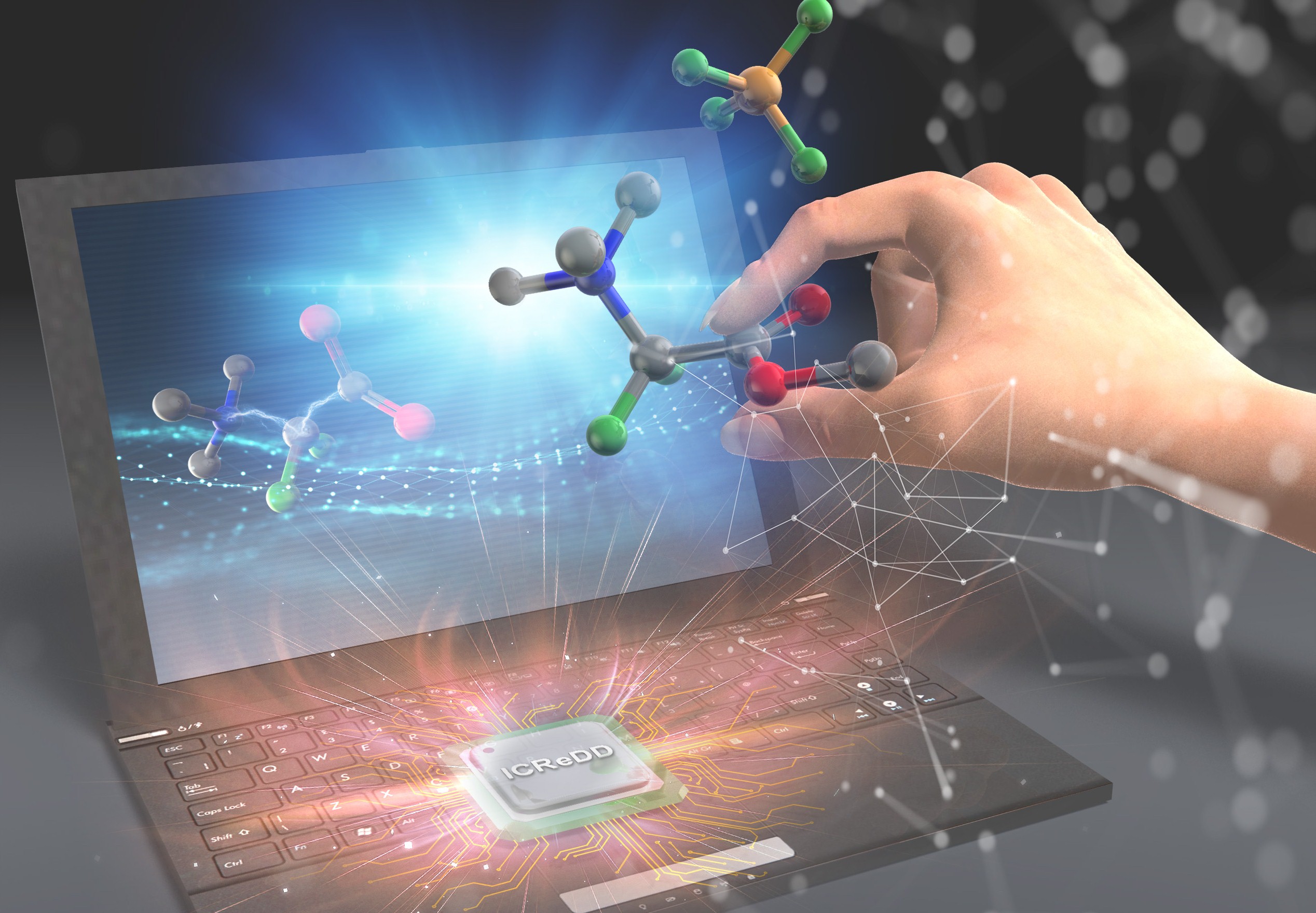
Artificial intelligence holds immense potential for rapidly mapping massive chemical datasets, accelerating drug discovery and materials development. AI's data processing capabilities can create expansive "chemical spaces" cataloging properties of billions of molecular configurations. This could enable a "Google Maps" for chemistry – an ambitious undertaking that may transform R&D.
For years, scientists have utilized digital chemical environments visualizing vast molecular data as interactive maps. Compounds are projected based on similarity, with proximity denoting shared attributes like thermal/light reactivity. Platforms like ChemMaps chart over 166 billion organic molecules up to 17 atoms, with public 3D navigation. It incorporates nearly 1 million drug and toxic compounds, plus biological testing data.
However powerful, these spaces only cover limited datasets. Comprehensively mapping all feasible molecular arrangements and their stability/traits poses a monumental challenge. At a recent American Chemical Society conference, researchers proposed modern AI's computing power could achieve this goal, ultimately expediting new molecule discovery.
Pharmaceutical development's long timelines – 10-15 years – involve target identification, optimizing compositions, trials, etc. Computer-assisted prediction of compound properties and effects has quickened the pace. But these techniques rely on existing data. AI utilizes vast biomedical datasets for synthesis versus predefined principles. With processing capable of comparing 10^60 molecules, AI could establish an expansive chemical space, slashing development to days or weeks.
A kindred tool, AlphaFold, predicts any protein structure. Yet chemical spaces remain arduous given data limitations. Much information on irrelevant compounds or techniques isn't published. However, MIT scientists are pioneering an AI-powered chemical space. It identifies molecular catalysts, evaluates stability by manipulating atoms/substitutions to populate surroundings with analogs. Property-specific subspaces also emerge, like toxic compounds clustering together. Solar energy storage and greenhouse gas conversion materials have been uncovered via this methodology.
In summary, AI's data synthesis potential could enable unprecedented chemical maps, accelerating R&D. Compiling exhaustive molecular configurations and attributes once seemed implausible. But AI computing power may realize this audacious goal and usher in a new era of accelerated materials and drug breakthroughs.
Share with friends:
Write and read comments can only authorized users
Last news