2024-04-03
AI identifies 5 types of Heart Failure to Predict risk and Treatment
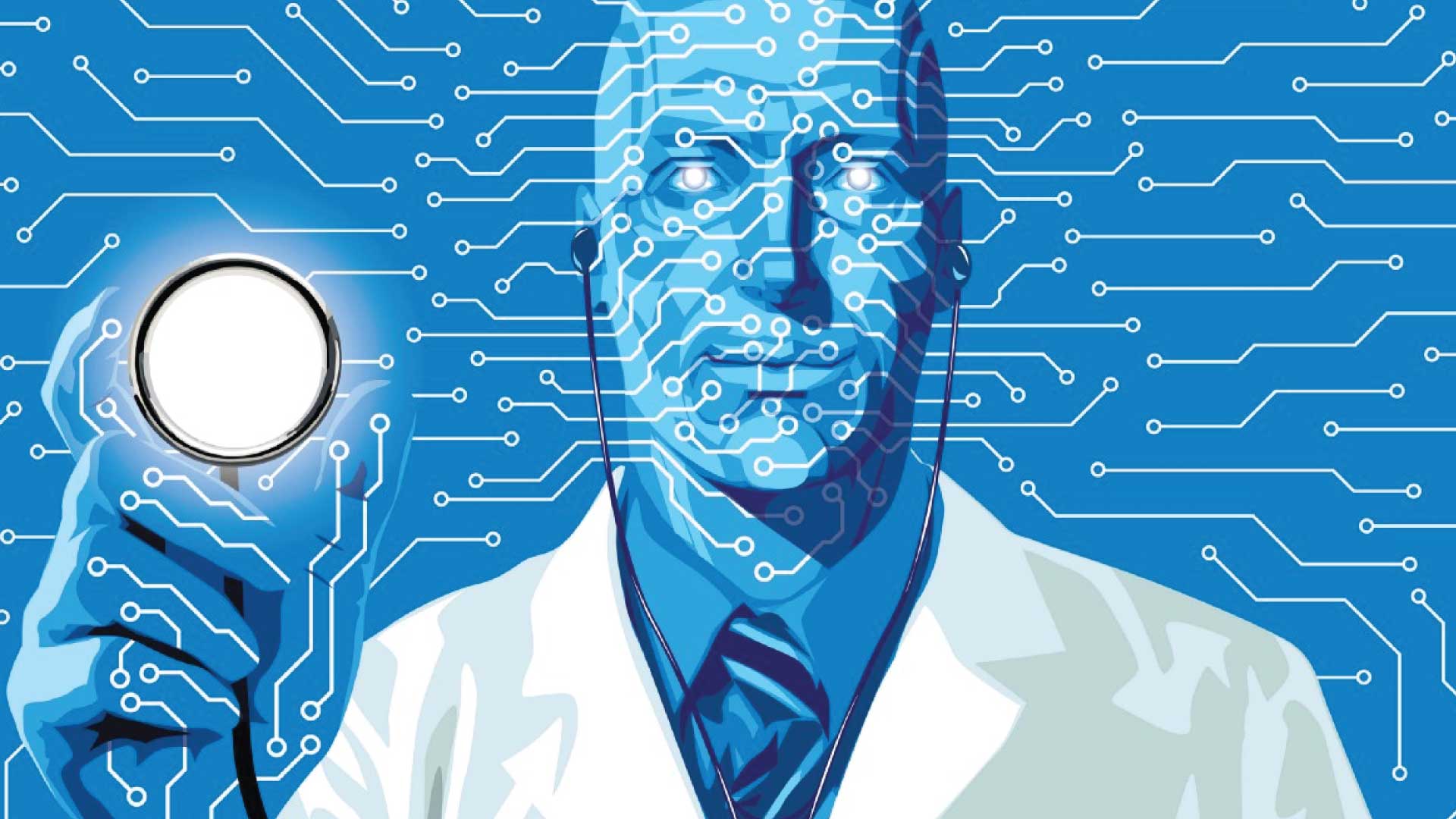
Unlocking new insights into heart failure, researchers from University College London leverage machine learning to redefine treatment strategies. In a groundbreaking study published in The Lancet Digital Health, Amitava Banerjee and colleagues utilize four machine learning models to classify heart failure patients into five distinct subtypes, transcending traditional classifications based solely on left ventricular ejection fraction (LVEF).
Drawing upon a comprehensive dataset of over 300,000 patients' electronic medical records spanning two decades, the researchers sought to enhance the understanding of heart failure prognosis and tailor treatment approaches to individual patients' needs. By integrating diverse factors such as age, symptoms, comorbidities, medications, and health parameters, the machine learning models identified five subtypes with unique characteristics.
- Early Starters: Young individuals with minimal risk factors.
- Late Start: Older patients with few medications and prevalent cardiovascular diseases.
- Associated with Atrial Fibrillation: Individuals with irregular heart rhythms or heart valve diseases.
- Metabolic: Overweight patients with average risk factors but low cardiovascular diseases.
- Cardiometabolic: Overweight individuals with numerous medications, high risk factors, and prevalent cardiovascular diseases.
Crucially, the study unveils significant variations in mortality risk among the identified subtypes. Patients categorized as "Associated with Atrial Fibrillation" exhibit the highest mortality risk within one year of diagnosis (61%), followed by "Late Start" (46%), "Cardiometabolic" (37%), "Early Starters" (20%), and "Metabolic" (11%). These findings underscore the potential of personalized treatment strategies tailored to specific subtypes of heart failure.
The implications of this research extend beyond academia, offering tangible benefits for clinical practice. With the development of an application based on the machine learning approach, healthcare providers gain a powerful tool for accurately determining patients' subtypes and optimizing treatment decisions. By embracing a more nuanced understanding of heart failure, clinicians can deliver targeted interventions and potentially improve patient outcomes.
The study represents a pivotal step towards precision medicine in heart failure management, empowering healthcare professionals to navigate the complexities of the disease with greater precision and efficacy. As the field of machine learning continues to advance, further refinements in subtype classification and treatment optimization are anticipated, heralding a new era in cardiovascular care.
Stay informed about the latest advancements in heart failure research and personalized treatment strategies by exploring The Lancet Digital Health today.
Share with friends:
Write and read comments can only authorized users
Last news