2024-06-16
AI-powered simulation platform RoboCasa aims to revolutionize robot training
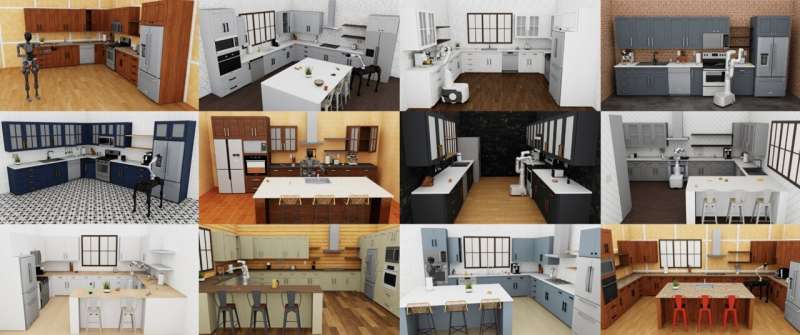
In a significant leap forward for robotics research, scientists from the University of Texas at Austin and NVIDIA Research have unveiled RoboCasa, an innovative large-scale simulation framework designed to train generalist robots for a wide array of everyday tasks. This groundbreaking platform, set to be presented at the Robotics: Science and Systems 2024 conference, represents a crucial step towards developing more capable and versatile robotic systems.
The advent of RoboCasa comes at a time when artificial intelligence has made remarkable strides in fields such as natural language processing and computer vision, largely due to the availability of massive datasets. However, the robotics domain has lagged behind, hampered by the challenges of acquiring sufficient training data for robot control and planning algorithms. RoboCasa aims to bridge this gap by providing a rich, diverse, and highly realistic simulated environment for robot learning.
Led by Yuke Zhu, the research team drew inspiration from recent AI advancements to create a platform capable of supplying high-quality simulation data for training robotics foundation models. "Recent progress in AI has been largely propelled by training large models on massive data sources," Zhu explained. "Inspired by these advancements, we seek to develop foundation models for generally capable robots that can perform various everyday tasks."
RoboCasa builds upon the team's previous work on RoboSuite, expanding it into a more comprehensive and sophisticated simulation framework. The platform boasts an impressive array of features, including:
- Thousands of 3D scenes with over 150 types of everyday objects
- Dozens of furniture items and electrical appliances
- 100 carefully designed tasks for algorithm training
- High-quality human demonstrations for these tasks
- Methods for generating effective trajectories and motions
One of the key innovations in RoboCasa is the use of generative AI tools to create diverse object assets, scenes, and tasks. This approach significantly enhances the realism and variety of the simulated environments, making them more representative of real-world scenarios. Additionally, the platform supports various robot hardware platforms, increasing its versatility and applicability across different robotic systems.
The researchers have also compiled an extensive dataset of over 100,000 trajectories, providing a robust foundation for model training. This wealth of data is crucial for developing more sophisticated and capable robotic systems.
In their initial experiments, Zhu and his colleagues made two exciting discoveries. First, they observed a clear scaling trend: as the size of the machine-generated training datasets increased, the model's performance showed steady improvement. This finding suggests that larger and more diverse datasets could lead to even more capable robotic systems in the future.
Second, the team found that combining simulation data with real-world data resulted in enhanced robot performance in real-world tasks. This hybrid approach could potentially bridge the gap between simulated environments and real-world applications, addressing one of the persistent challenges in robotics research.
The open-source nature of RoboCasa, available on GitHub, invites collaboration and experimentation from the broader robotics community. This accessibility could accelerate progress in the field, allowing researchers worldwide to build upon and improve the platform.
Looking ahead, Zhu and his team have ambitious plans for RoboCasa's future development. They aim to incorporate more advanced generative AI methods to further expand their simulations, capturing an even greater variety of human-centered environments. From homes and factories to offices, the goal is to create increasingly realistic and diverse virtual spaces for robot training.
Furthermore, the researchers plan to develop improved algorithms that can better leverage simulation data to build more robust and generalizable robotics systems for real-world applications. This ongoing work could potentially lead to significant breakthroughs in robot capabilities and adaptability.
As the field of robotics continues to evolve, platforms like RoboCasa play a crucial role in pushing the boundaries of what's possible. By providing a rich, diverse, and highly realistic training environment, RoboCasa could accelerate the development of more capable and versatile robots, bringing us closer to a future where robots can seamlessly assist humans in a wide range of everyday tasks.
The introduction of RoboCasa marks an important milestone in robotics research, offering a powerful tool for training the next generation of intelligent robots. As the platform continues to evolve and improve, it promises to play a pivotal role in shaping the future of robotics and artificial intelligence.
Share with friends:
Write and read comments can only authorized users