2024-04-03
Training algorithms for safe actions in an unfamiliar environment
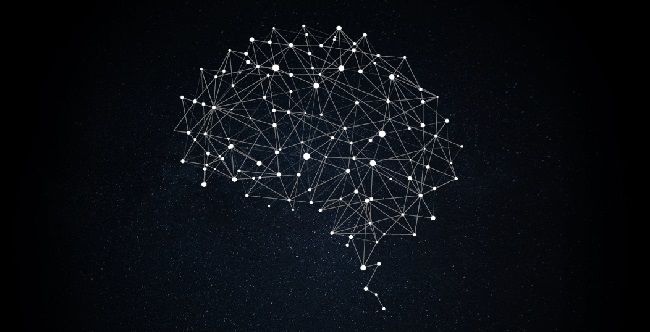
Children who are just learning to walk may walk too fast and fall or collide with furniture. However, this element of causality teaches them invaluable information about how their bodies move in space so that they can avoid falling in the future.
Machines learn in much the same way as humans, including learning from their mistakes. However, for many machines, such as autonomous cars and power systems, learning in real conditions is a challenge. With the development and spread of machine learning, there is growing interest in its application in very complex, security-critical autonomous systems. However, the prospects of these technologies are constrained by the security risks inherent in the learning process and not only.
A new research paper refutes the idea that an unlimited number of tests are needed to teach safe actions in an unfamiliar environment. The paper, published in the IEEE Transactions on Automatic Control journal, presents a new approach that provides training in safe actions with complete confidence, while maintaining a balance between optimality, dangerous situations and rapid recognition of unsafe actions.
"Machine learning usually looks for the most optimal solution, which can lead to an increase in the number of errors along the way. This is problematic when an error can mean a collision with a wall," explained Juan Andres Bazerk, associate professor of electrical and computer engineering at the Swanson School of Engineering, who led the study with associate professor Enrique Mallada from Johns Hopkins University. "In this study, we show that teaching safe politics is fundamentally different from teaching optimal politics, and that it can be done separately and effectively."
The research team conducted research in two different scenarios to illustrate their concept. They have created an algorithm that detects all unsafe actions within a limited number of rounds. The team also solved the problem of finding the optimal policy for the Markov decision process (MDP) with almost certain limitations.
Their analysis highlighted the trade-off between the time needed to detect unsafe activities in the underlying MDP and the level of exposure to unsafe events. MDP is useful because it provides a mathematical framework for modeling decision-making in situations where the results are partly random and partly under the control of the decision maker.
To confirm their theoretical conclusions, the researchers conducted a simulation that confirmed the identified trade-offs. These results also showed that enabling security restrictions can speed up the learning process.
"This study refutes the prevailing opinion that an unlimited number of tests are required to teach safe actions," Bazerk said. - Our results show that by effectively managing the tradeoffs between optimality, exposure to unsafe events and detection time, we can achieve guaranteed safety without an infinite number of studies. This has significant implications for robotics, autonomous systems, artificial intelligence and more."
Share with friends:
Write and read comments can only authorized users
Last news