2024-02-16
Cognitive Pilot has solved a difficult task in training neural networks
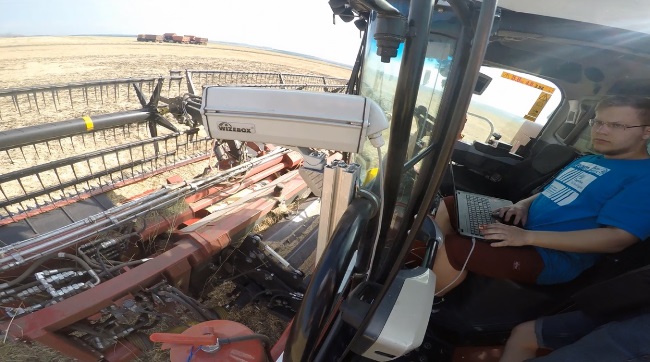
Russian AI firm Cognitive Pilot has developed an innovative technology called Cognitive Smart OoD to automatically identify uncommon real-world cases that pose risks for autonomous vehicles. By detecting rare out-of-distribution data like obscured traffic lights or animals, their approach aims to boost training and safety for self-driving cars and transport.
Most autopilot systems are trained on only common driving datasets which leaves corner cases unaddressed. But accounting for every uncommon and dangerous situation that could randomly appear is critical for true fail-safe operation.
"It's like radium mining, it can take tens of man-years," said Gennady Savitsky, Head of Unmanned Transport at Cognitive Pilot, describing traditional manual annotation. "It is simply unrealistic for a human to make a high-quality selection manually."
The startup's Cognitive Smart OoD solution leverages neural networks and statistical methods to accurately label rare objects and events within massive video streams. This allows efficiently pinpointing rail crossing malfunctions, wayward farm animals, and other outliers that developers previously struggled discovering themselves.
The automated data mining innovation has been implemented in the company’s commercial self-driving units across agriculture and public transit. CEO Olga Uskova touts it as a huge breakthrough addressing a longstanding obstacle facing the autonomous vehicle field.
"In almost all cases our autopilot system is now able to clearly understand the situation and respond adequately to ensure maximum traffic safety," Uskova said. "This is an extremely big event in the AI market."
By exponentially amplifying data variability fed into training pipelines, the startup aims to massively improve reliability beyond levels feasible manually. Safer autonomy then unlocks unmanned trams and tractor-trailers among commercial prospects.
The advancement builds on Cognitive Pilot's prior automation developments around representative data tagging itself. With both common and rare sample handling now autonomous, the company is positioned to efficiently scale datasets covering the sprawling complexity of real-world transportation.ф
Share with friends:
Write and read comments can only authorized users
Last news