2024-05-01
Concordia student develops AI model to help detect suicidal ideation through speech
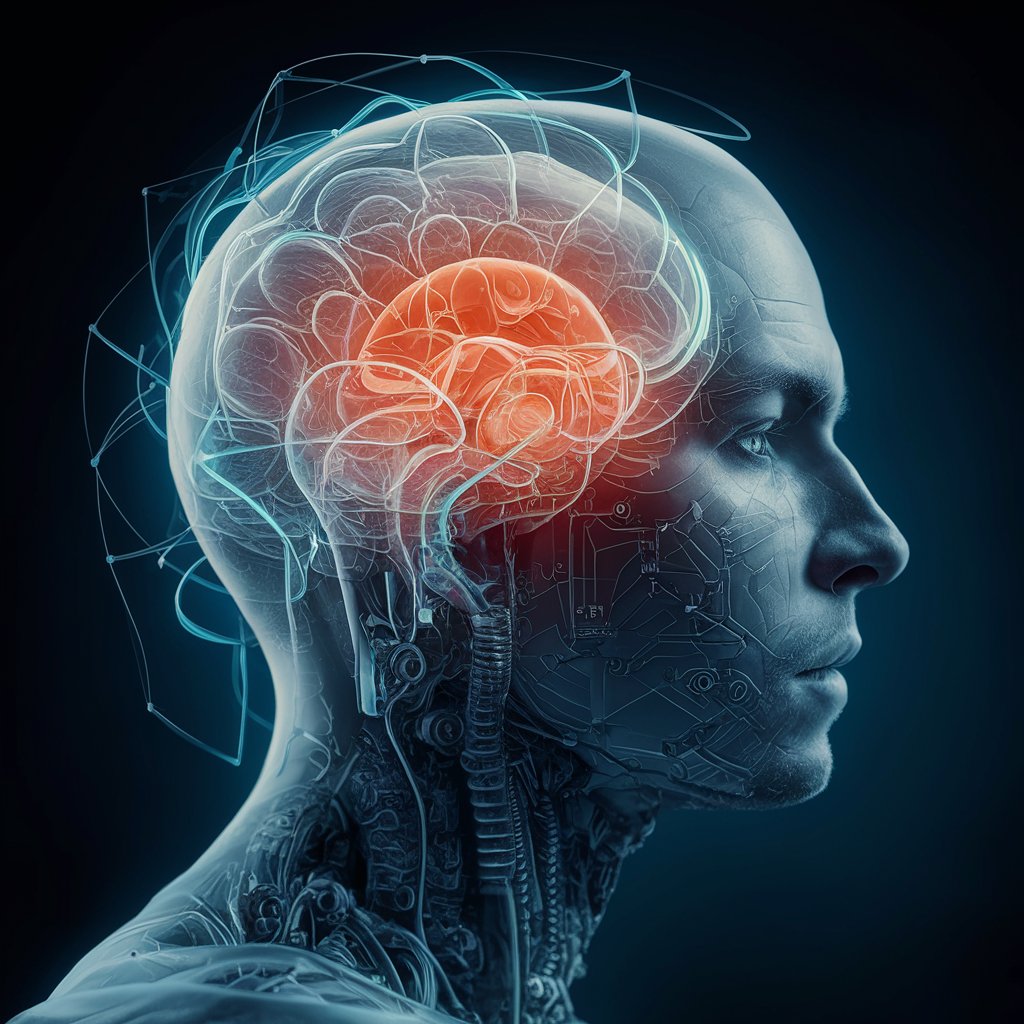
Speech is a vital cue in detecting suicidal ideation and understanding the mental and emotional state of those experiencing it. Suicide hotline counselors undergo extensive training to quickly analyze variations in callers' speech patterns to provide the most appropriate intervention during a crisis.
However, even the most skilled counselors can miss subtle vocal signals that could inform better response strategies. To enhance support for those at risk, Alaa Nfissi, a Ph.D. student at Concordia University, has developed an innovative speech emotion recognition (SER) model leveraging artificial intelligence tools.
The model, which analyzes and codes waveform modulations in callers' voices, shows promise in improving real-time suicide risk assessment and monitoring. Nfissi's research was recently published and presented at the 2024 IEEE 18th International Conference on Semantic Computing (ICSC), where it received the prestigious Best Student Paper Award.
"Traditionally, SER was done manually by trained psychologists who would annotate speech signals, which requires high levels of time and expertise," Nfissi explains. "Our deep learning model automatically extracts speech features that are relevant to emotion recognition."
A member of Concordia's Centre for Research and Intervention on Suicide, Ethical Issues and End-of-Life Practices (CRISE), Nfissi built his model using a database of actual calls to suicide hotlines, merged with recordings from a diverse range of actors expressing specific emotions. Each segment was meticulously annotated to reflect four emotional states: angry, neutral, sad, or fearful/concerned/worried.
Employing deep learning architectures like neural networks and gated recurrent units, Nfissi's model can process variable-length data sequences, extracting local and time-dependent features that convey emotional nuances through a time process. This approach overcomes limitations of older models that required fixed-length segments.
The results are compelling: Nfissi's model accurately recognized fearful/concerned/worried emotions 82% of the time, neutral 78%, sad 77%, and angry 72%. Its performance was particularly impressive with professionally recorded segments, correctly identifying emotions between 78% (sad) and 100% (angry) of the time.
For Nfissi, whose work involved an in-depth study of suicide hotline interventions, the project is deeply personal. "Many of these people are suffering, and sometimes just a simple intervention from a counselor can help a lot. However, not all counselors are trained the same way, and some may need more time to process and understand the emotions of the caller."
He envisions his model being integrated into a real-time dashboard for counselors, providing valuable insights to guide appropriate intervention strategies. "This will hopefully ensure that the intervention will help them and ultimately prevent a suicide."
Professor Nizar Bouguila from Concordia's Institute for Information Systems and Engineering co-authored the paper, along with Wassim Bouachir from Université TÉLUQ and CRISE, and Brian Mishara from UQÀM and CRISE.
As AI continues to advance, Nfissi's work represents a promising step toward leveraging technology to save lives and provide better support for those grappling with suicidal thoughts – a crucial application in the field of mental health and suicide prevention.
Share with friends:
Write and read comments can only authorized users