2024-06-28
DIVID: Columbia engineering's new frontier in detecting AI-generated videos
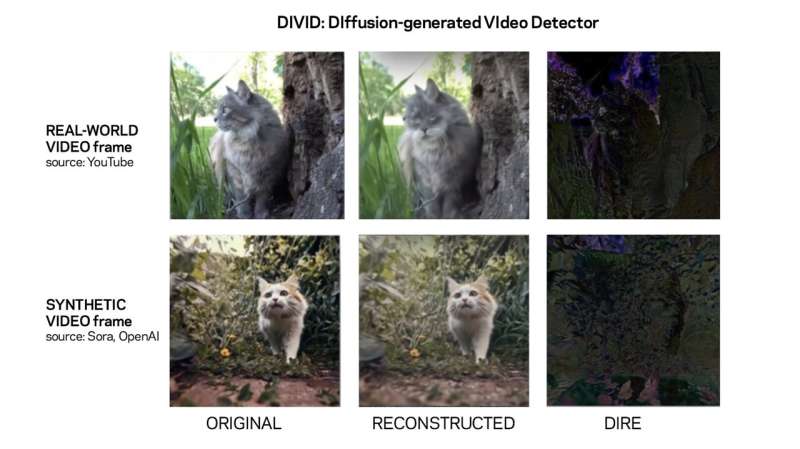
In an era where artificial intelligence (AI) is blurring the lines between reality and fabrication, a team of researchers at Columbia Engineering has developed a groundbreaking tool that promises to restore trust in video content. Named DIVID (DIffusion-generated VIdeo Detector), this innovative technology represents a significant leap forward in the ongoing battle against increasingly sophisticated AI-generated videos.
The Rising Threat of AI-Generated Videos
The urgency of this development is underscored by recent events that highlight the potential for devastating consequences when AI-generated content is weaponized. Earlier this year, a multinational corporation fell victim to a $25 million fraud scheme where criminals used AI to create convincingly realistic videos of the company's CFO and other executives. This incident serves as a stark reminder of the growing capabilities of AI in mimicking human appearance and behavior, and the pressing need for robust detection methods.
As AI video generation tools like OpenAI's Sora, Runway Gen-2, and Pika continue to advance, the challenge of distinguishing between authentic and synthetic videos has become increasingly complex. These new-generation tools utilize diffusion models, a sophisticated AI technique that gradually refines random noise into clear, lifelike images and videos. The result is content so realistic that it can fool not only human observers but also existing detection systems.
DIVID: A New Approach to Video Authentication
Led by Computer Science Professor Junfeng Yang, the Columbia Engineering team has developed DIVID as a response to this escalating threat. DIVID builds upon the team's earlier work on Raidar, a tool designed to detect AI-generated text. The key insight driving both Raidar and DIVID is that AI-generated content often exhibits characteristics that other AI systems consider high-quality, resulting in fewer edits or alterations when processed.
DIVID's approach to video authentication is based on a technique called DIRE (DIffusion Reconstruction Error). This method measures the difference between an input video and its reconstructed version using a pretrained diffusion model. The underlying hypothesis is that AI-generated videos will show minimal differences when reconstructed, as they already conform to the statistical norms learned by the AI model. In contrast, human-created videos are more likely to deviate from these norms, resulting in greater differences upon reconstruction.
Impressive Accuracy and Potential Applications
The effectiveness of DIVID is remarkable, with the team reporting detection accuracy of up to 93.7% for videos from their benchmark dataset. This dataset included diffusion-generated videos from popular AI tools such as Stable Vision Diffusion, Sora, Pika, and Gen-2, representing a broad spectrum of current AI video generation capabilities.
While DIVID is currently available as a command-line tool for developers, its potential applications are vast. The researchers envision integrating DIVID as a plugin for video conferencing platforms like Zoom, enabling real-time detection of deepfake calls. There are also plans to develop a website or browser plugin, making this powerful detection tool accessible to ordinary users.
The Broader Implications
The development of DIVID comes at a critical juncture in the evolution of digital media. As AI-generated content becomes increasingly prevalent and difficult to distinguish from authentic material, the potential for misinformation, fraud, and manipulation grows exponentially. Tools like DIVID are essential not only for preventing financial crimes but also for maintaining the integrity of public discourse, protecting individuals from identity theft, and preserving trust in digital communications.
Moreover, the open-sourcing of DIVID's code and datasets by the Columbia Engineering team represents a significant contribution to the broader scientific community. By making these resources available, the researchers are enabling further development and refinement of AI detection techniques, fostering a collaborative approach to addressing this global challenge.
Looking Ahead
As promising as DIVID is, the researchers acknowledge that this is an ongoing battle. The team is already working on improving the framework to handle a wider variety of synthetic videos from open-source video generation tools. They are also using DIVID to collect and analyze more videos, continually expanding their dataset to enhance the tool's effectiveness.
The rapid advancement of AI video generation technologies means that detection methods must evolve just as quickly. The success of DIVID demonstrates the potential for innovative approaches in staying ahead of malicious actors who seek to exploit these technologies.
The development of DIVID by Columbia Engineering researchers marks a significant milestone in the field of AI-generated content detection. As our digital landscape continues to evolve, tools like DIVID will play a crucial role in maintaining the authenticity and trustworthiness of visual information. While the challenge of AI-generated content is far from solved, DIVID represents a powerful step forward in our ability to navigate an increasingly complex digital reality.
As we move forward, the ongoing refinement and widespread adoption of such detection tools will be critical in preserving the integrity of our digital interactions and safeguarding against the potential misuse of AI technologies. The work of Professor Yang and his team at Columbia Engineering serves as a beacon of hope in this ongoing technological arms race, reminding us that human ingenuity remains our strongest asset in the face of evolving digital threats.
Share with friends:
Write and read comments can only authorized users