2024-04-01
Drone Detects Sosnovsky's Hogweed
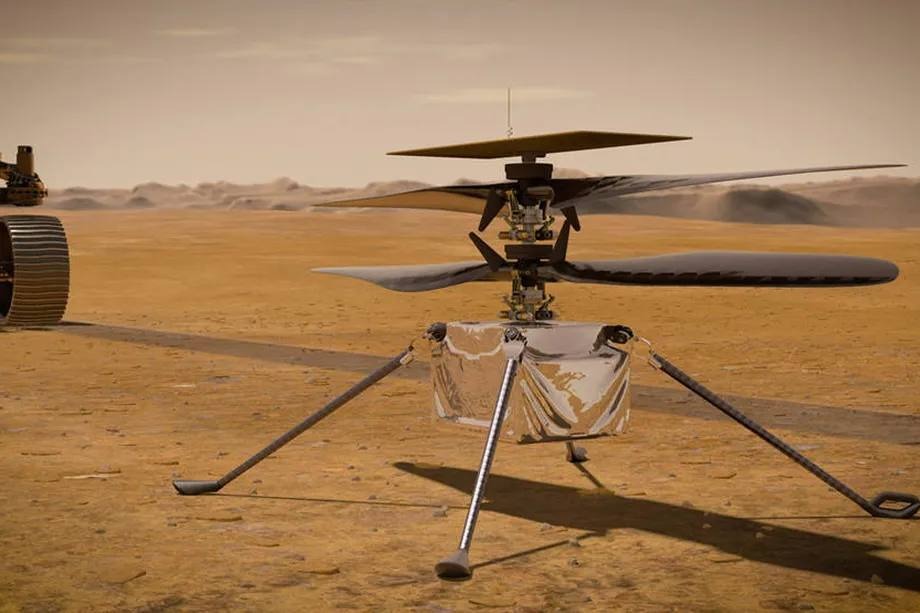
In the ongoing battle against invasive species, Russian engineers have unleashed a powerful new weapon: a drone equipped with cutting-edge technology to detect and eradicate Sosnovsky's hogweed (Heracleum sosnowskyi). This innovative solution, developed and tested with remarkable success, marks a significant milestone in the fight against this notorious plant scourge.
Sosnovsky's hogweed stands as one of the most notorious and pervasive invasive species, wreaking havoc across Russia and beyond. Originating from the Caucasus region, its introduction to other parts of the former USSR in the mid-20th century precipitated a relentless march of proliferation, defying attempts at containment and control. Despite decades of efforts, the spread of hogweed continues unabated, posing a persistent threat to ecosystems and agricultural landscapes alike.
Central to the challenge of combating hogweed is its tenacity and prolific seed dispersal, which renders conventional detection and mapping methods ineffective. Traditionally, manual surveys or costly aerial reconnaissance have been employed to identify and target hogweed-infested areas. However, these approaches are labor-intensive, time-consuming, and often yield suboptimal results.
Enter the innovative solution devised by Skoltech engineers under the leadership of Andrey Somov: a drone-based detection system powered by advanced artificial intelligence. Leveraging the DJI Matrice 200 quadcopter as the hardware platform and the NVIDIA Jetson Nano single-board computer equipped with a potent video accelerator, this system represents a paradigm shift in invasive species management.
At the heart of the drone's capabilities lies a sophisticated convolutional neural network (CNN), meticulously trained to identify Sosnovsky's hogweed in aerial imagery. Through the process of semantic segmentation, the CNN analyzes drone-captured frames, precisely delineating areas inhabited by hogweed for subsequent eradication.
To optimize performance, the engineers evaluated three state-of-the-art CNN architectures—U-Net, SegNet, and RefineNet—against their dataset meticulously assembled from drone footage captured in the Moscow region. After rigorous training and testing, the U-Net-based network emerged as the top performer, achieving impressive detection rates at a speed of 0.7 frames per second.
The successful implementation of the drone-based detection system underscores the transformative potential of unmanned aerial vehicles in ecological conservation and agricultural management. With its ability to autonomously survey vast expanses of land and accurately pinpoint invasive species, this technology offers a cost-effective and scalable solution to the ongoing threat posed by Sosnovsky's hogweed.
Moreover, the implications extend beyond hogweed detection, as similar drone-based approaches have been employed in diverse contexts, from identifying cotton fields and forest vegetation to combating illicit crops like coca bushes. As the field of drone technology continues to evolve, the possibilities for leveraging unmanned aerial vehicles in environmental stewardship and conservation efforts are boundless.
In conclusion, the development of the drone-based hogweed detection system represents a pivotal advancement in the fight against invasive species, demonstrating the power of innovation and collaboration in addressing complex ecological challenges. With its ability to swiftly and accurately identify and eradicate hogweed-infested areas, this technology offers hope for a more resilient and sustainable future for ecosystems and communities alike.
Share with friends:
Write and read comments can only authorized users