2024-04-03
Unlocking Drone Mastery: MIT's Liquid Neural Networks Lead the Way!
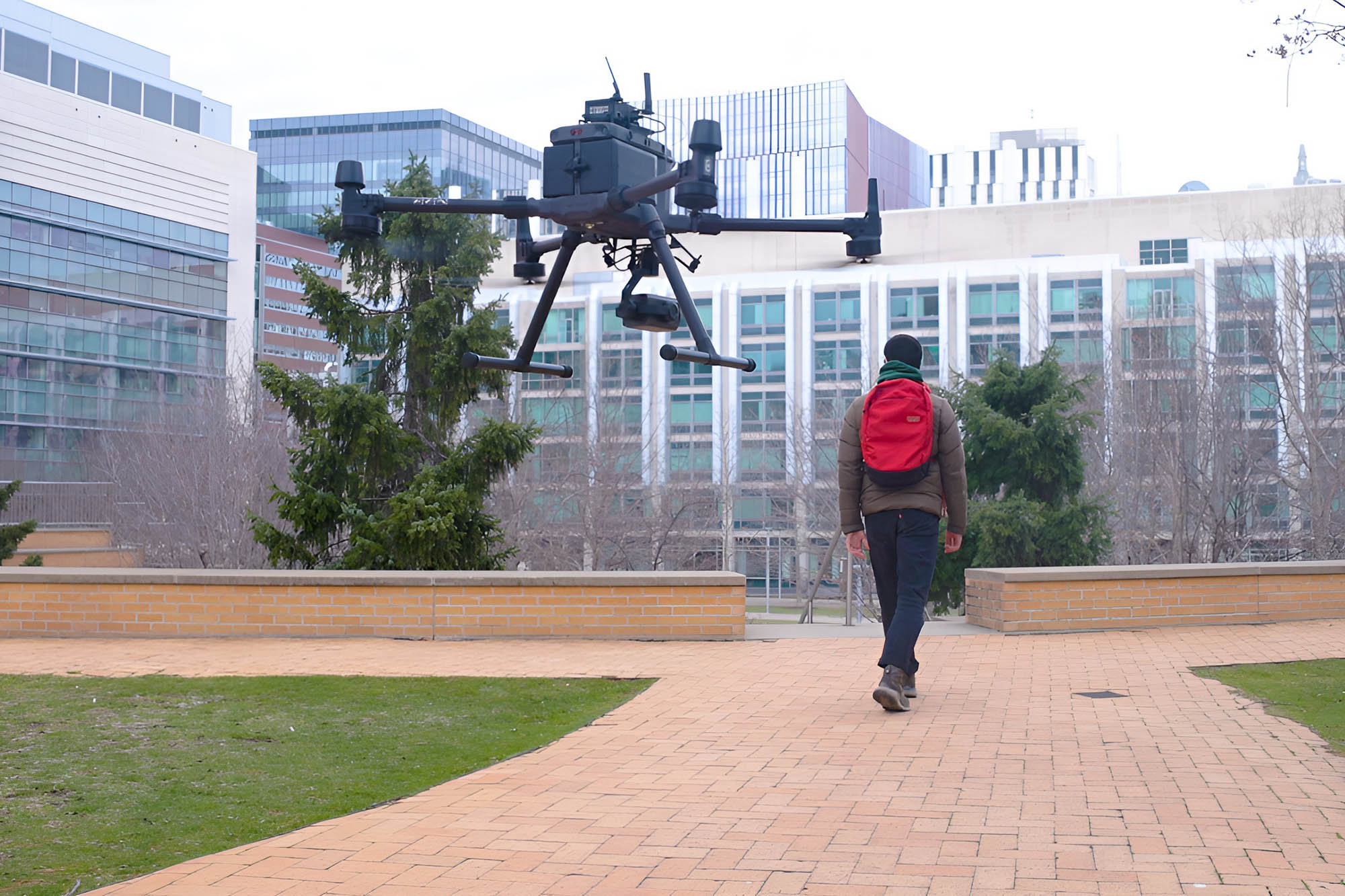
MIT's Computer Science and Artificial Intelligence Laboratory (CSAIL) has unveiled a groundbreaking approach to drone navigation, revolutionizing the way drones master vision-based fly-to-target tasks in intricate and unfamiliar environments. Through the innovative use of liquid neural networks, MIT researchers have paved the way for drones to adapt and excel in diverse landscapes and scenarios.
The liquid neural networks developed by MIT CSAIL's team exhibit remarkable adaptability, particularly in navigating through challenging environments such as forests, urban landscapes, and areas with added noise, rotation, and occlusion. Surpassing many state-of-the-art counterparts, these networks offer promising potential for real-world applications including search and rescue missions, delivery services, and wildlife monitoring.
Daniela Rus, Director of CSAIL and Andrew (1956) and Erna Viterbi Professor of Electrical Engineering and Computer Science at MIT, emphasizes the transformative impact of their approach, stating, "Our learning-based control approach lays the groundwork for solving problems arising from training in one environment and deploying in a completely distinct environment without additional training." The adaptability of these algorithms holds vast implications for various fields, from medical diagnosis to autonomous driving.
MIT CSAIL's liquid neural networks are designed to capture the causal structure of tasks from high-dimensional, unstructured data, such as pixel inputs from drone-mounted cameras. By extracting crucial task aspects and filtering out irrelevant features, these networks facilitate seamless transfer of navigation skills to new environments.
In their research, the team observed promising indications of the liquid networks' ability to address a critical weakness in deep machine-learning systems. Unlike traditional neural networks that learn solely during the training phase, liquid neural networks possess parameters that can adapt over time, rendering them resilient to unexpected or noisy data.
Through a series of quadrotor closed-loop control experiments, MIT CSAIL's drones demonstrated exceptional performance in diverse scenarios, including target tracking, navigating through dynamic environments, and executing multi-step loops between objects. These experiments underscored the drones' capacity to learn from limited expert data and generalize tasks to new environments.
MIT CSAIL envisions a future where drones equipped with liquid neural networks streamline autonomous operations, making deployment more efficient, cost-effective, and reliable. From environmental monitoring to package delivery and autonomous vehicle navigation, liquid neural networks offer unprecedented potential in reshaping the capabilities of aerial drones.
Share with friends:
Write and read comments can only authorized users
Last news