2024-03-25
Household robots with "common sense" to overcome disruptions
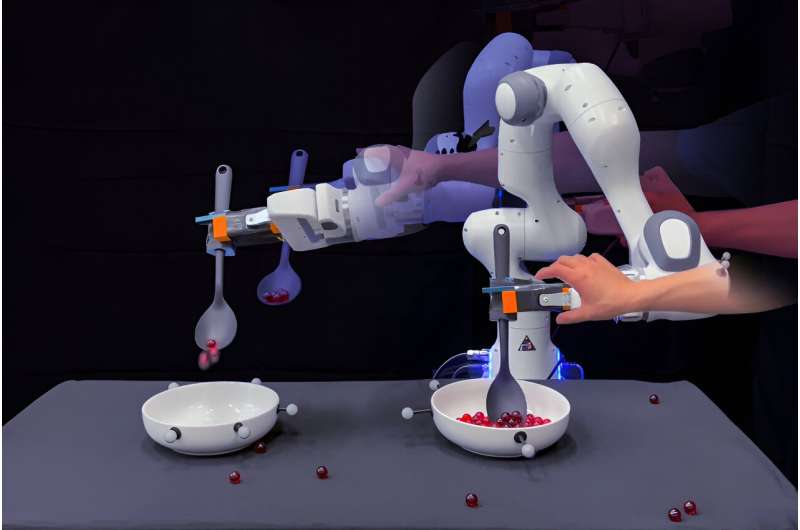
In the ever-evolving quest to bring robots into our homes, researchers at MIT have taken a significant step forward by endowing these mechanical helpers with a crucial trait: common sense. A team led by graduate student Yanwei Wang from the Department of Electrical Engineering and Computer Science (EECS) has developed an innovative method that connects robot motion data with the "common sense knowledge" of large language models (LLMs), enabling household robots to logically parse tasks into subtasks and adapt to disruptions without having to start over from scratch.
From wiping up spills to serving food, robots are increasingly being trained to carry out intricate household chores through a process called imitation learning, where they mimic the motions a human physically guides them through. However, as the MIT researchers discovered, blindly mimicking these motions can lead to compounding errors if the robot encounters unexpected obstacles or nudges along the way.
"Imitation learning is a mainstream approach enabling household robots. But if a robot is blindly mimicking a human's motion trajectories, tiny errors can accumulate and eventually derail the rest of the execution," explains Wang. "With our method, a robot can self-correct execution errors and improve overall task success."
The key to their breakthrough lies in the integration of large language models (LLMs) – deep learning systems trained on vast text data – with the physical motion trajectories demonstrated to the robots. By prompting an LLM to generate a logical sequence of subtasks for a given household chore, such as "reach," "scoop," "transport," and "pour" for scooping marbles from one bowl into another, the researchers developed an algorithm to automatically connect these natural language labels with the corresponding physical coordinates or image data of the robot's motions.
This "grounding" algorithm essentially acts as a translator, facilitating a dialogue between the robot's actions in the physical world and the LLM's understanding of the subtasks involved, allowing the robot to identify which stage of the task it is currently executing.
"The grounding classifier facilitates this dialogue between what the robot is doing in the physical space and what the LLM knows about the subtasks, and the constraints you have to pay attention to within each subtask," Wang elaborates.
In experiments with a robotic arm trained on the task of scooping marbles from one bowl into another, the researchers demonstrated the remarkable capabilities of their approach. As the robot carried out the task autonomously, they intentionally pushed, nudged, and disrupted its motions, knocking marbles off its spoon at various points.
Rather than halting and starting from the beginning or blindly continuing without the required objects, the robot leveraged its newfound "common sense" to self-correct and complete each subtask successfully before proceeding to the next. It would ensure it had scooped up marbles before attempting to transport them, adapting its actions in real-time based on the situational context.
"With our method, when the robot is making mistakes, we don't need to ask humans to program or give extra demonstrations of how to recover from failures," Wang says, highlighting the significant reduction in engineering effort achieved by their approach.
The implications of this breakthrough extend far beyond the humble task of scooping marbles. As researchers continue to collect training data for household robots through teleoperation systems, Wang's algorithm can convert that data into robust, adaptable robot behaviors capable of tackling complex tasks despite external disruptions.
"That's super exciting because there's a huge effort now toward training household robots with data collected on teleoperation systems. Our algorithm can now convert that training data into robust robot behavior that can do complex tasks, despite external perturbations," Wang affirms.
This infusion of "common sense" into household robotics paves the way for a future where our mechanical assistants can seamlessly navigate the unpredictable realities of our homes, adjusting their actions on the fly and overcoming obstacles without the need for constant human intervention or reprogramming.
As the field of household robotics continues to evolve, the work of Wang and his colleagues at MIT represents a significant stride toward realizing the dream of truly capable and adaptable robotic companions in our homes, ushering in a new era of convenience and efficiency while pushing the boundaries of what is possible in human-robot collaboration.
Share with friends:
Write and read comments can only authorized users