2023-12-21
LETI has come up with a new reaction model to create a "chemical" neural network
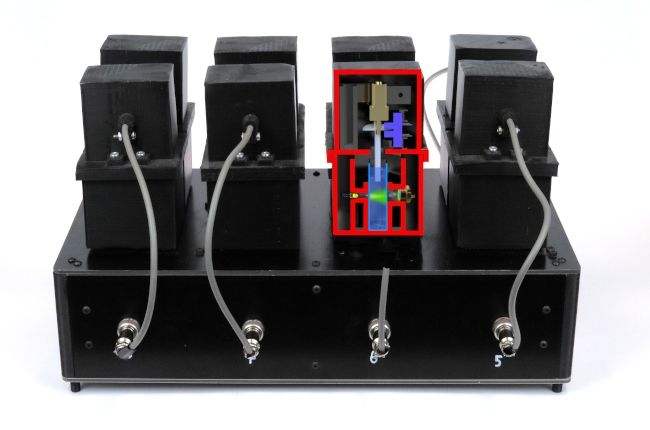
Scientists at St. Petersburg State Technical University "LETI" (SPbSETU "LETI") have developed a mathematical model to simulate chemical reactions that could enable a new type of computer architecture inspired by the human brain. As artificial intelligence (AI) systems like neural networks transform various industries, some researchers believe unconventional computing hardware based on physical chemistry rather than silicon chips could overcome limitations in processing power, speed, cost, and energy use.
The proposed model accurately describes the nonlinear dynamics of the famous Belousov-Zhabotinsky (BZ) reaction, an oscillating chemical process where mixing certain reagents causes visible color changes from red to blue in a cyclic fashion. Prior models failed to capture the complex and chaotic fluctuations observed experimentally, but the new equations match the empirical data more closely. Controlling and predicting BZ reactions computationally opens possibilities for building "chemical neural networks."
According to Timur Karimov, Associate Professor of Computer-Aided Design Systems at SPbSETU LETI, “We have developed a mathematical model that describes the chemical processes of the well-known Belousov-Jabotinsky reaction...The ability to control such a reaction and accurately simulate it can be used to create a new type of computer.”
Conventional Computers Constrained by Hardware
Today's AI achievements depend on advanced algorithms but also require massive computing power and data storage from expensive, energy-hungry hardware. As companies race to improve system performance, they keep reaching physical limitations in silicon-based microchips. Chemical computing provides an alternative paradigm that encodes information directly in the states of chemical solutions instead of digital bits. Networks of interacting chemical reactions could replicate key aspects of neural processing, avoiding bottlenecks facing digital devices.
Harnessing the Belousov-Zhabotinsky Reaction
Discovered independently in the 1950s and 60s by Russian biophysicist Anatol Zhabotinsky and Ukrainian chemist Boris Belousov, the BZ reaction remains a seminal example of non-equilibrium thermodynamics. Its most striking feature is the spontaneous emergence of nonlinear oscillations, where concentrations of intermediates periodically spike and crash. The reaction can maintain this cycling for hours, with parameters sensitive to initial concentrations and external perturbations.
BZ systems display rich spatiotemporal patterns, far from equilibrium. Their seeming lifelike behavior provides analogies to biological phenomena like heartbeats or nerve impulses. The chaotic dynamics and self-organization make BZ media an appealing platform for unconventional computing. Networks of coupled BZ reactions could replicate neural mechanisms like memory formation, learning, and information storage more efficiently than silicon circuits.
Improved Mathematical Modeling with New Equations
Harnessing BZ reactions for chemical computers requires mathematical models that accurately capture the intricate workings of the complex reactions. While previous models successfully described some qualitative features, the LETI researchers developed new differential equations better matching empirical observations.
As Ekaterina Kopets, Assistant Professor of CAD Systems, explains, “Experiments with an array of connected test tubes carried out by foreign colleagues show that such a reservoir really has a memory.” By tweaking parameters, scientists can encode information to control the dynamics. The model equations will facilitate designing and optimizing "chemical neural networks" computationally without relying solely on time-consuming lab tests.
Insights for Developing Chemical Neural Networks
The proposed reservoir computing framework utilizes instrumented BZ reactions as a “chemical brain” where external neural networks then analyze the emerging spatiotemporal patterns. Kopets notes, “If you compare one tube to an individual neuron, then the connection between the tubes is a synaptic connection. Thus, an artificial neural network is formed from test tubes with a chemical reaction.”
As a hypothetical example, mixing reagents in specified tubes could represent a binary input pattern. As reactions propagate through the array, sensors would capture the dynamical response, and machine learning algorithms would interpret the readout. Chemical computers built along these lines could outperform digital devices for selected applications by harnessing intrinsic physical computations.
Simulation Before Fabrication Using New Model
Rather than wrestling with practical difficulties handling wet chemistry, the improved model equations developed at LETI enable studying hypothetical chemical computers through computer simulation first. Lead researcher Artur Karimov argues hybrid systems combining microfluidics with machine learning algorithms offer promising alternative computing paradigms. “Experiments with real reagents are time-consuming and suffer from poor repeatability, so the question of how best to organize a chemical computer is optimally solved by modeling.”
Looking Ahead
The LETI researchers published their mathematical modeling study in Chaos, Solitons and Fractals, a cutting-edge nonlinear science journal. More work remains to design and optimize virtual chemical computers, as well as fabricate prototypical microfluidic chips capable of demonstrating the promised performance advantages compared to digital circuits. If chemical neural networks can be competitive for applications like pattern recognition or controlling autonomous robots, this bio-inspired approach could supplement current silicon technologies. Beyond innovations in AI software algorithms, creating new physical computing devices tailored for neural network workloads will likely play a pivotal role in overcoming intrinsic limits constraining further progress.
Share with friends:
Write and read comments can only authorized users
Last news