2024-06-12
MIT's novel "policy composition" AI teaches robots to master any tool
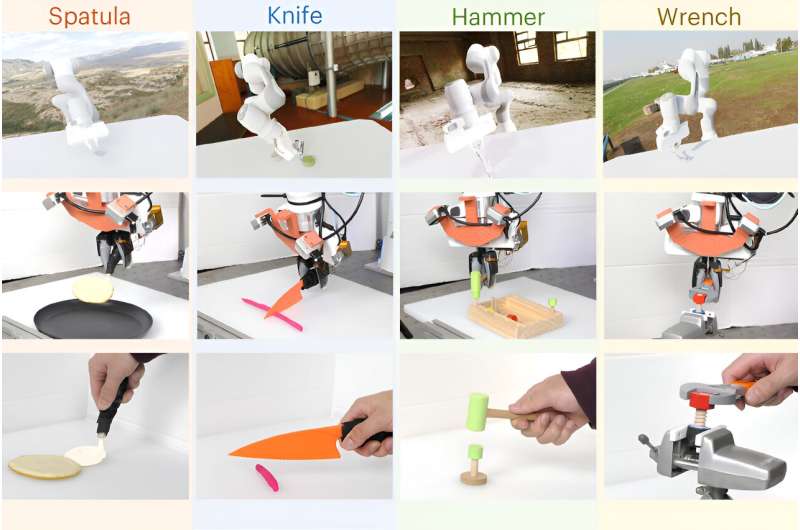
In a breakthrough that could pave the way for more versatile robots, MIT researchers have developed a novel technique to train robots on an incredibly diverse range of tool-use tasks by combining multiple data sources into a single powerful AI system. The new method, dubbed Policy Composition or "PoCo", represents a significant step towards creating truly general-purpose robots adept at everything from repairing home appliances to assembling products on factory floors.
The core challenge PoCo overcomes is the vast heterogeneity across existing robotic datasets. While warehouses, research labs, and other facilities may have amassed terabytes of training data demonstrating specific tasks like packing boxes or operating certain machinery, this data exists in siloed modalities and environments. Integrating such scattered data resources to produce a capable, generalized robotic intelligence has remained an immense hurdle.
"It's a chicken-and-egg problem," explains Lirui Wang, an MIT electrical engineering graduate student who led the development of PoCo. "We need highly deployable general robots to collect all this diverse data in the first place. Leveraging all the heterogeneous data out there is key to training these robots, similar to how AI models like ChatGPT ingest massive multimodal datasets."
PoCo's innovative solution is to use diffusion models - a powerful type of generative AI traditionally used for media synthesis tasks like image generation. Instead of creating images, the researchers' diffusion models learn policies or "strategies" detailing the precise trajectories and movements a robot should take to complete a task using the equipment at hand, whether a hammer, wrench, or kitchen spatula.
By training separate diffusion models on individual narrowly-scoped datasets covering different tasks, environments, and data modalities, the team can extract specialized policies from each. PoCo then combines and refines these individual policies into a generalized composite policy that melds the strengths of each constituent dataset.
"One policy might pick up dexterity from human video demonstrations, while another provides better generalization from a robotics simulation," explains Wang. "PoCo lets us mix-and-match their advantages into policies for entirely new tasks the robot was never explicitly trained on."
In simulations and real-world tests with robotic arms tackling repair and object manipulation tasks, PoCo demonstrated over 20% improved performance compared to traditional training methods limited to single datasets. The AI system excelled not just at known tasks but quickly generalized its tool skills to master novel tasks its training had never covered.
Perhaps most promisingly, the team showed PoCo could continually expand its repertoire simply by integrating new datasets representing new tasks, domains or modalities - no retraining required.
"We're combining the best of internet data, simulations, and real-world recordings into powerfully general robot control policies," says MIT's Russ Tedrake, senior author on the PoCo research. "It's an important step towards finally realizing robots with practical, multifaceted tool use skills that can quickly adapt to boundless applications."
As PoCo and similar techniques continue advancing, the team envisions robotic assistants equally capable of repairing home appliances, assembling intricate products, or even practicing dexterous surgery - maximizing their potential by consuming all available data, not just what falls in a narrow silo. The age of flexible, general-purpose robots may finally be within reach.
Share with friends:
Write and read comments can only authorized users
Last news