2024-07-18
MIT researchers develop breakthrough algorithm for safer AI-controlled robots
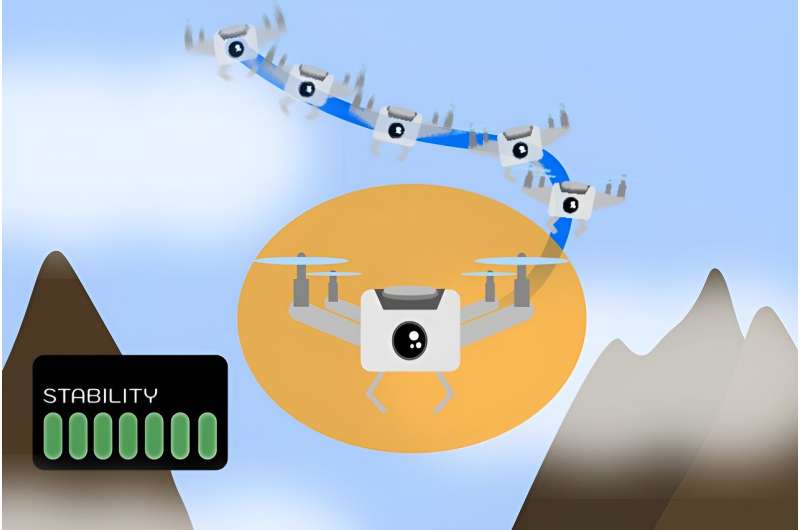
In a groundbreaking development at the intersection of artificial intelligence and robotics, researchers from MIT's Computer Science and Artificial Intelligence Laboratory (CSAIL) have unveiled a new algorithm that could revolutionize the safety and reliability of AI-controlled machines. This innovative approach addresses one of the most significant challenges in the field: ensuring the stable and safe operation of robots powered by complex neural networks.
The advent of neural networks has dramatically transformed the landscape of robotic control systems, enabling more adaptive and efficient machines. However, this increased sophistication comes at a cost – the complexity of these brain-like machine-learning systems makes it exceedingly difficult to guarantee that a neural network-powered robot will safely accomplish its assigned tasks.
Traditionally, engineers have relied on Lyapunov functions to verify the safety and stability of control systems. These mathematical tools provide a way to ensure that unsafe or unstable situations, associated with higher values in the Lyapunov function, will never occur. However, applying these techniques to robots controlled by neural networks has proven challenging, with previous approaches struggling to scale effectively to more complex machines.
The MIT team, led by Ph.D. student Lujie Yang and Toyota Research Institute researcher Hongkai Dai, has developed new techniques that rigorously certify Lyapunov calculations in more elaborate systems. Their algorithm efficiently searches for and verifies a Lyapunov function, providing a stability guarantee for the system. This breakthrough could potentially enable safer deployment of robots and autonomous vehicles, including aircraft and spacecraft.
Yang explains the significance of their work: "We've seen some impressive empirical performances in AI-controlled machines like humanoids and robotic dogs, but these AI controllers lack the formal guarantees that are crucial for safety-critical systems. Our work bridges the gap between that level of performance from neural network controllers and the safety guarantees needed to deploy more complex neural network controllers in the real world."
The researchers' approach introduces a novel shortcut to the training and verification process. By generating cheaper counterexamples – such as adversarial data from sensors that could potentially confuse the controller – and then optimizing the robotic system to account for them, the team was able to create more robust and adaptable machines. This method enables the robots to operate safely in a wider range of conditions than previously possible.
Furthermore, the team developed a novel verification formulation that allows the use of a scalable neural network verifier called α,β-CROWN. This verifier provides rigorous worst-case scenario guarantees that go beyond the counterexamples, adding an extra layer of safety assurance.
To demonstrate the effectiveness of their algorithm, the team conducted several digital simulations. In one experiment, they successfully guided a quadrotor drone with lidar sensors to a stable hover position in a two-dimensional environment, using only the limited environmental information provided by the lidar sensors. Additional experiments showed the stable operation of an inverted pendulum and a path-tracking vehicle over a wider range of conditions than previously achievable.
The potential applications of this stability approach are vast and could significantly impact various fields where safety is paramount. From ensuring smoother rides in autonomous vehicles to enhancing the reliability of drones used for delivery or terrain mapping, the implications are far-reaching. The researchers suggest that their techniques could even find applications beyond robotics, potentially benefiting fields such as biomedicine and industrial processing.
While this new method represents a significant leap forward in terms of scalability, the researchers acknowledge that there is still work to be done. Future research directions include improving performance in systems with higher dimensions, accounting for more diverse types of sensor data, and providing stability guarantees for systems operating in uncertain environments subject to disturbances.
The team also aims to apply their method to optimization problems, focusing on minimizing the time and distance a robot needs to complete a task while maintaining stability. Ultimately, they hope to extend their technique to more complex machines like humanoids and other real-world robots that need to maintain stability while interacting with their surroundings.
As AI and robotics continue to advance and integrate into various aspects of our lives, the work of Yang and her colleagues at MIT CSAIL represents a crucial step towards ensuring that these powerful technologies can be deployed safely and reliably. By bridging the gap between performance and safety guarantees, this research paves the way for a future where AI-controlled machines can operate with greater autonomy and security in increasingly complex environments.
Share with friends:
Write and read comments can only authorized users