2024-05-12
MIT researchers unlock the potential of shape-shifting "slime robots"
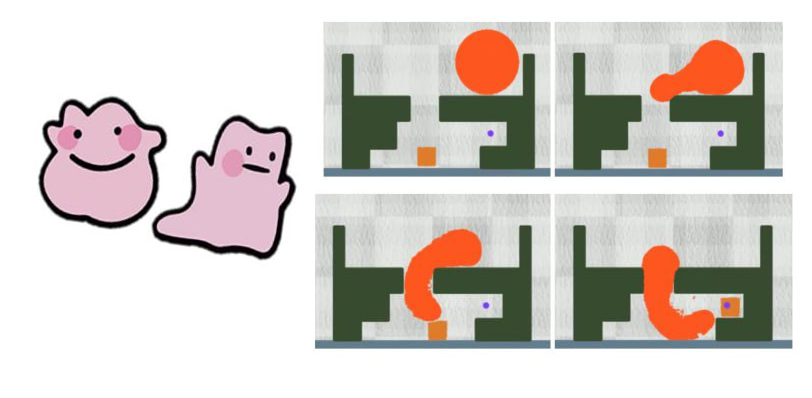
In a groundbreaking development that sounds like something straight out of a science fiction movie, researchers at the Massachusetts Institute of Technology (MIT) have made significant strides in controlling shape-shifting "slime robots" – squishy, reconfigurable robots that can seamlessly alter their morphology to squeeze through narrow spaces or adopt entirely new forms.
While the concept of a robot that can change its shape at will may seem like a distant dream, the team at MIT's Computer Science and Artificial Intelligence Laboratory (CSAIL) has brought us one step closer to making it a reality. Their latest research, published on the arXiv preprint server, introduces a novel control algorithm that can autonomously learn how to move, stretch, and shape a reconfigurable robot to complete specific tasks, even when those tasks require the robot to change its morphology multiple times.
Imagine a scenario where a slime-like robot is deployed inside the human body to remove an unwanted item, effortlessly navigating through the intricate pathways by adapting its shape as needed. Or envision a wearable device that can morph its form to provide customized support and comfort. These are just a glimpse of the potential applications that could be unlocked by this groundbreaking research.
The challenge of controlling a soft, shape-shifting robot is daunting – without joints, limbs, or fingers to manipulate, traditional control methods fall short. "When people think about soft robots, they tend to think about robots that are elastic, but return to their original shape. Our robot is like slime and can actually change its morphology. It is very striking that our method worked so well because we are dealing with something very new," explains Boyuan Chen, an electrical engineering and computer science graduate student at MIT and co-author of the paper.
At the core of their approach is a reinforcement learning algorithm that takes a coarse-to-fine methodology. Rather than attempting to control each tiny muscle individually, the algorithm begins by learning to control groups of adjacent muscles that work together. Then, after exploring the space of possible actions, it drills down into finer detail to optimize the action plan it has learned.
To enable this, the researchers treat the robot's action space – how it can move in a certain area – as an image. Their machine-learning model uses images of the robot's environment to generate a 2D action space, which includes the robot and the area around it. By understanding the relationships between nearby action points, much like nearby pixels in an image form coherent shapes, the algorithm can effectively control the robot's motion.
To test their approach, the researchers developed a simulation environment called DittoGym, featuring eight tasks that evaluate a reconfigurable robot's ability to dynamically change shape. From elongating and curving its body to weave around obstacles to mimicking letters of the alphabet, their algorithm outperformed baseline methods and proved to be the only technique suitable for completing multistage tasks that required several shape changes.
"Our task selection in DittoGym follows both generic reinforcement learning benchmark design principles and the specific needs of reconfigurable robots. Each task is designed to represent certain properties that we deem important, such as the capability to navigate through long-horizon explorations, the ability to analyze the environment, and interact with external objects," explains Suning Huang, an undergraduate student at Tsinghua University in China and the lead author of the paper.
While the deployment of shape-shifting robots in real-world applications may still be years away, the MIT researchers' work has ignited a new wave of excitement and inspiration in the field. "We have a stronger correlation between action points that are closer to each other, and I think that is key to making this work so well," notes Chen, hinting at the potential for this approach to be leveraged for other complex control problems.
As the world eagerly awaits the next chapter in the development of these "slime robots," one thing is certain: the research being conducted at MIT's CSAIL is pushing the boundaries of what was once thought impossible, ushering in a new era of soft, reconfigurable robotics that could revolutionize industries from healthcare to wearable technology and beyond.
Share with friends:
Write and read comments can only authorized users
Last news