2024-05-06
Researchers tackle machine learning's reproducibility crisis
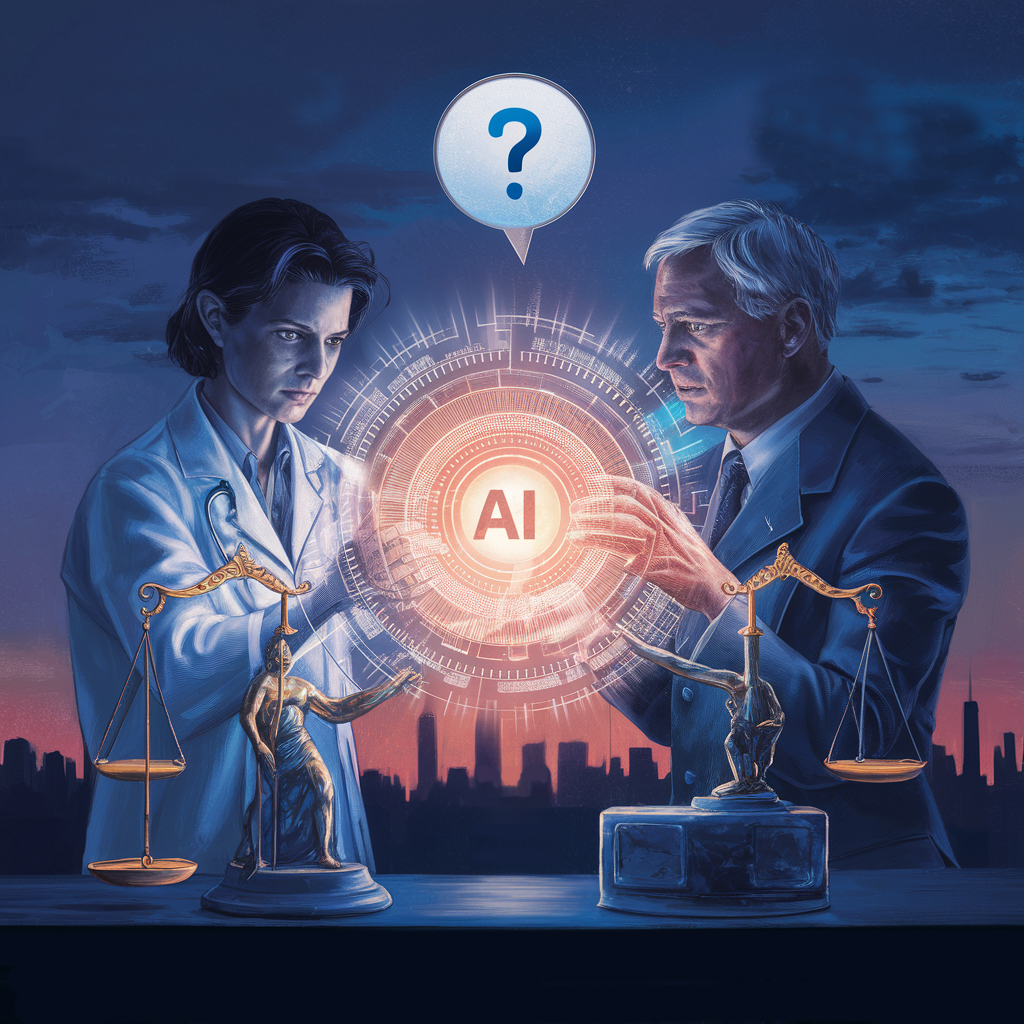
Artificial intelligence and machine learning have incredible potential to drive scientific breakthroughs, from helping doctors detect diseases earlier to guiding policymakers away from disastrous decisions. However, a growing body of evidence reveals that the cutting-edge machine learning techniques being rapidly adopted across virtually every scientific field are plagued by serious flaws and lack robust standards. Thousands of published papers have been implicated, representing a looming "reproducibility crisis" that could be even more damaging than the replication crisis that rocked psychology over a decade ago.
A team of 19 interdisciplinary researchers led by Princeton University computer scientists Arvind Narayanan and Sayash Kapoor is taking direct aim at this smoldering crisis by publishing new guidelines for the responsible use of machine learning methods in scientific research. Their work, detailed in a paper in the journal Science Advances, establishes a simple framework and checklist to ensure the integrity and reproducibility of studies utilizing machine learning algorithms and models.
"When we graduate from traditional statistical methods to machine learning methods, there are a vastly greater number of ways to shoot oneself in the foot," warns Narayanan, who directs Princeton's Center for Information Technology Policy. "If we don't have an intervention to improve our scientific standards and reporting standards when it comes to machine learning-based science, we risk not just one discipline but many different scientific disciplines rediscovering these crises one after another."
The lack of universal standards protecting the credibility of machine learning research across fields represents an existential threat to the entire scientific enterprise, which depends on the ability to independently validate and build upon published results. Without that core reproducibility, the entire system risks collapse.
"This is a systematic problem with systematic solutions," asserts Kapoor, the graduate student who organized the interdisciplinary effort to establish consensus-driven guidelines. The new checklist centers on enforcing transparency, calling on researchers to provide detailed documentation of their machine learning models, code, data, hardware configurations, experimental designs, research goals, and limitations.
While adopting more stringent reporting standards may slow the publication pace for individual studies, the authors argue this short-term tradeoff will pay massive dividends by improving research quality and accelerating the overall rate of discovery and innovation. "What we ultimately care about is the pace of scientific progress," says sociologist Emily Cantrell, one of the lead authors pursuing her PhD at Princeton. "By making sure the papers that get published are of high quality and that they're a solid base for future papers to build on, that potentially then speeds up the pace of scientific progress. Focusing on scientific progress itself and not just getting papers out the door is really where our emphasis should be."
The consequences of ignoring this machine learning reproducibility crisis could be catastrophic and far-reaching, the researchers warn. "At the collective level, it's just a major time sink," Kapoor states. "That time costs money. And that money, once wasted, could have catastrophic downstream effects, limiting the kinds of science that attract funding and investment, tanking ventures that are inadvertently built on faulty science, and discouraging countless numbers of young researchers."
To uphold scientific integrity and keep "honest people honest," as Narayanan puts it, the authors intend for their guidelines to be widely adopted by researchers improving their own practices, by peer reviewers evaluating papers, and by journals establishing publication requirements. "The scientific literature, especially in applied machine learning research, is full of avoidable errors," Narayanan says. "And we want to help people."
By taking this reproducibility crisis head-on and enacting robust, consensus-driven standards, this interdisciplinary group aims to clear a path for machine learning to fulfill its remarkable potential to propel scientific progress across all fields. The credibility of the entire research enterprise depends on it.
Share with friends:
Write and read comments can only authorized users
Last news