2024-04-02
NVIDIA Trains Robotic Arm Using Simulation for Faster and Efficient Learning
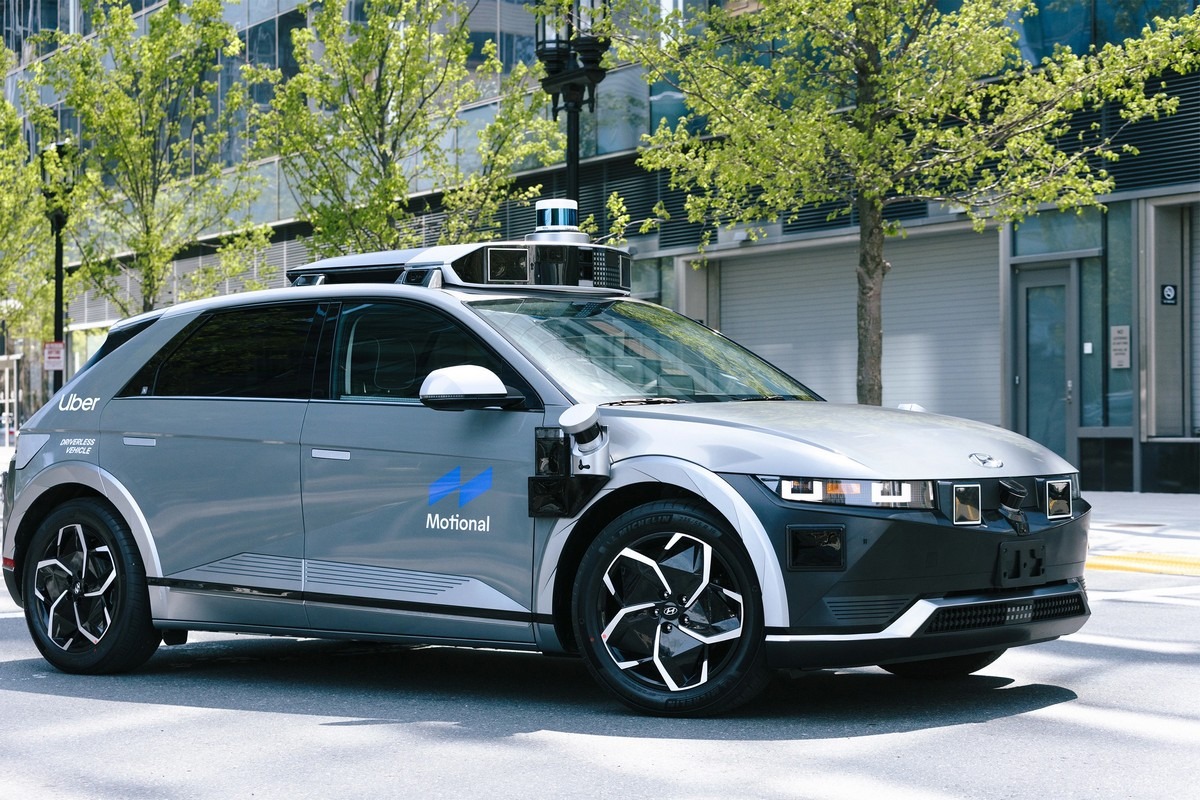
Robotic arms are complex machines that require advanced training methods to perform tasks efficiently. Traditional methods such as pre-programmed movements and deep reinforcement learning are not suitable for controlling robotic arms. To overcome this challenge, NVIDIA used its Isaac Gym RL robotics simulator to train the Allegro Hand, a lightweight anthropomorphic robotic arm with three serial cameras attached, as part of its DeXtreme project.
The Isaac simulator can run more than 10,000 times faster simulations than the real world, while obeying the laws of physics. With the help of Isaac Gym, NVIDIA was able to teach the Allegro Hand to manipulate the cube and match given target positions, orientations, or poses. NVIDIA's neural network brain learned to do all this in a simulation, and then the team transplanted it to control the robot in the real world.
In addition to its comprehensive Isaac Gym simulation environment, NVIDIA used its PhysX simulator, which simulates the world on the GPU, which remains in GPU memory while the deep learning control policy network is trained, to train the arm. Simulation training provides a number of benefits for robotics. In addition to NVIDIA's ability to run simulations much faster than in the real world, the robot's hardware is prone to breakage after extended use. According to NVIDIA, the team working on the arm often had to stop to fix the robotic arm, such as tightening screws, changing ribbon cables, and resting the arm to cool down after prolonged use. This hinders the learning that a robot needs in the real world.
To train the robot's neural network, NVIDIA's Omniverse Replicator generated about five million frames of synthetic data, meaning the NVIDIA team didn't have to use real images. In the NVIDIA training method, the neural network is trained using a technique called domain randomization, which changes the lighting and camera position to give the network more robust capabilities. All training was done on a single Omniverse OVX server, and the system can train a good policy in about 32 hours. According to NVIDIA, the robot will take 42 years to get the same experience in the real world.
The use of simulation for training robotic arms has revolutionized the way robots are trained for various tasks. With the help of NVIDIA's Isaac Gym and PhysX simulators, robots can be trained in a faster and more efficient way, reducing the need for real-world training. This technology has the potential to transform various industries, including manufacturing, logistics, and healthcare, by enabling robots to perform complex tasks with greater precision and speed. As the demand for robot workers continues to grow, the use of simulation for training robots will become increasingly important.
Share with friends:
Write and read comments can only authorized users
Last news