2023-11-20
Robotic system for assessing the mobility of stroke patients
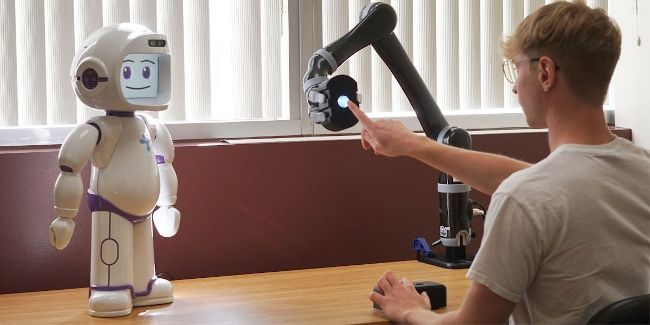
Researchers from the University of Southern California have developed a groundbreaking robotic system that aims to provide a more accurate assessment of how stroke survivors use their weak limbs during their recovery process. The system allows tracking of three-dimensional spatial information using a robotic manipulator and utilizes machine learning to process the collected data. By analyzing metrics such as the probability of using the hand, reaching time, and successful reaching, the system can determine the extent of "non-use of a limb," also known as "learned non-use." This valuable information can assist medical professionals in accurately evaluating a patient's progress in rehabilitation.
The innovative system, known as the Social-Assisting Robot (SAR), not only collects precise data but also motivates patients through instructions and encouragement during the procedure. The goal is to assess how the results of physical therapy transfer to real-life situations. This integration of quantitative data from a robotic arm and the motivation provided by a social robot creates a more accurate and motivating process for evaluating the condition of stroke patients.
During the study, 14 participants with a dominant right hand before their stroke were recruited. They were required to stretch out their hand and touch a device with touch sensors, fixed in the grip of the manipulator. Machine learning techniques were then employed to analyze the three dimensions and determine the "non-use of a limb" metric.
The participants faced a time limit to reach for the button, prompting an intuitive reaction to turn on the light. Even though they were aware they were being tested, they still needed to react quickly. This measurement enabled the researchers to observe patterns in hand usage and assess whether there was a preference for the stronger or weaker limb.
The team found that individuals who had experienced a chronic stroke exhibited high variability in hand usage and reaching time. This innovative method proved to be reliable over repeated sessions, and the participants rated it as easy to use. Furthermore, the participants stated that the system was safe and straightforward to interact with.
The differences in hand usage discovered during the study offer valuable insights that can help medical professionals better track a stroke patient's recovery. The system has the potential to be further personalized, as the team hopes to explore in future studies. Additionally, incorporating additional behavioral data, such as facial expressions and various types of tasks, could enhance the system's capabilities and overall effectiveness.
Share with friends:
Write and read comments can only authorized users
Last news