2024-04-13
The surprising impact of prompt variations on ChatGPT and AI models
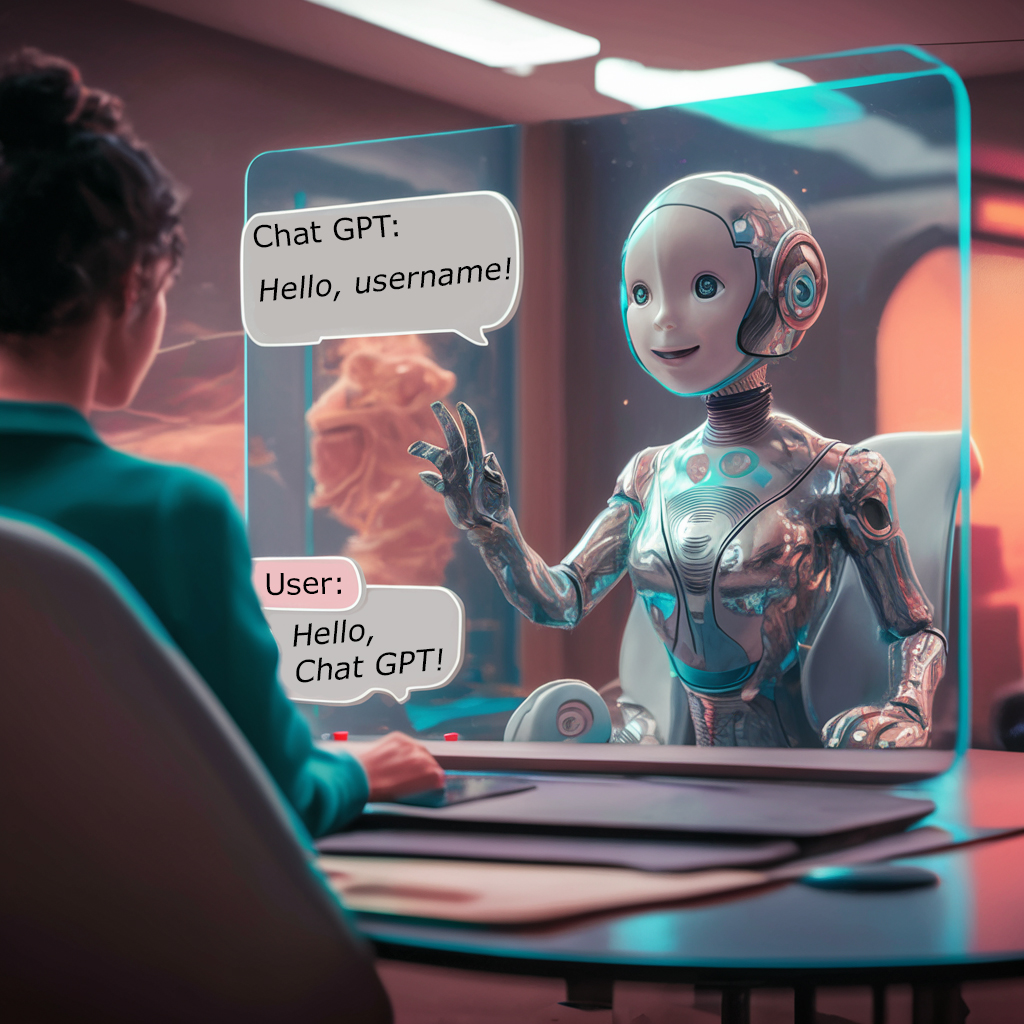
In the rapidly evolving world of artificial intelligence, large language models (LLMs) like ChatGPT have become ubiquitous, assisting researchers, analysts, and everyday users with a wide range of tasks. However, a recent study has unveiled a surprising finding: Subtle variations in the prompts given to these models can significantly impact their predictions and accuracy.
Researchers at the USC Information Sciences Institute (ISI) and the Viterbi School of Engineering set out to investigate the reliability of LLMs' responses to variations in prompts. Abel Salinas, a researcher at ISI, explained, “We are relying on these models for so many things, asking for output in certain formats, and wondering in the back of our heads, 'what effect do prompt variations or output formats actually have?' So we were excited to finally find out.”
The study, conducted by Salinas and Fred Morstatter, Research Assistant Professor of computer science at USC, delved into four categories of prompt variations. First, they examined the impact of requesting responses in specific output formats commonly used in data processing, such as lists or CSV files.
Second, they explored minor perturbations to the prompts themselves, such as adding extra spaces, incorporating polite phrases like "Thank you" or "Howdy!", or even offering monetary tips for "a perfect response."
Third, they investigated the use of "jailbreaks," techniques employed to bypass content filters when dealing with sensitive topics like hate speech detection, for example, asking the LLM to answer as if it were evil.
Across 11 benchmark text classification tasks, ranging from toxicity classification to humor and sarcasm detection, the researchers measured how often the LLM changed its response and the impact on its accuracy.
The findings were remarkable. Minor alterations in prompt structure and presentation could substantially impact LLM predictions. Adding specified output formats led to a minimum of 10% of predictions changing. Even introducing a space at the beginning or end of a prompt resulted in more than 500 prediction changes out of 11,000 instances.
Additionally, the researchers noted that certain prompt strategies, such as incentives or specific greetings, demonstrated marginal enhancements in accuracy, highlighting the nuanced relationship between prompt design and model behavior.
Interestingly, the researchers found no single formatting or perturbation method suited all tasks. The "No Specified Format" prompt achieved the highest overall accuracy, outperforming other variations by a full percentage point.
Salinas acknowledged the potential implications of these findings, stating, "We did find there were some formats or variations that led to worse accuracy, and for certain applications it's critical to have very high accuracy, so this could be helpful."
The reason behind this phenomenon is not entirely clear, but the researchers hypothesized that instances that change the most are those that are the most "confusing" to the LLM. They examined tasks where human annotators disagreed, potentially indicating confusion, and found some correlation between confusion and prediction changes, though not strong enough to be the sole explanatory factor.
Salinas posited that the relationship between the inputs the LLM is trained on and its subsequent behavior could play a role. If conversational elements like greetings are frequently associated with certain types of information during training, the model may learn to prioritize or interpret prompts differently based on those associations.
As the research community continues to explore the intricacies of LLMs, a major goal is to generate models that are resilient to these prompt variations, offering consistent and accurate responses across formatting changes, perturbations, and jailbreaks.
For now, Salinas offers a piece of advice for those prompting ChatGPT or other AI models: "The simplest finding is that keeping prompts as simple as possible seems to give the best results overall."
This study highlights the nuanced and complex nature of AI model behavior, emphasizing the need for continued research and understanding to unlock the full potential of these powerful tools while mitigating potential biases or inconsistencies.
Share with friends:
Write and read comments can only authorized users
Last news