2024-05-21
UT Austin researchers develop framework to train AI on corrupted images
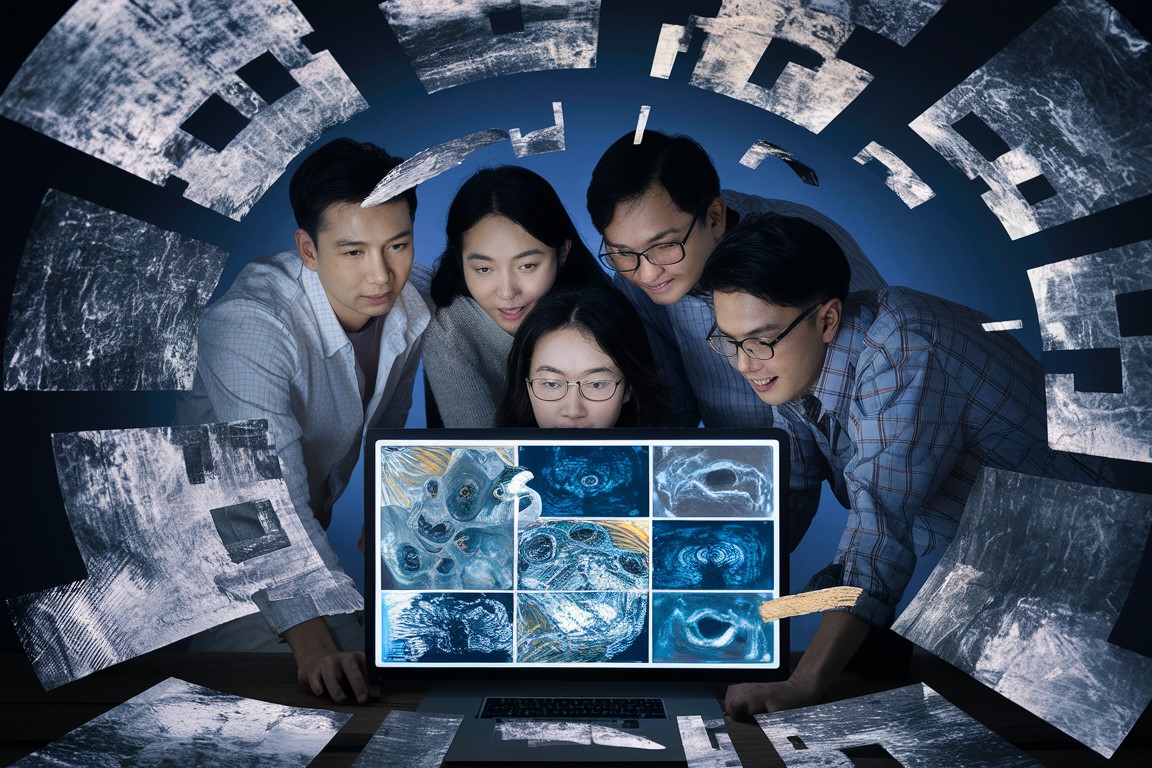
In a breakthrough that could reshape how powerful AI models are trained, researchers at The University of Texas at Austin have developed a novel framework called Ambient Diffusion that allows AI systems to learn from image data corrupted beyond recognition. This innovative approach aims to address growing concerns around memorization and copyright infringement haunting popular text-to-image generative AI like DALL-E, Midjourney and Stable Diffusion.
These cutting-edge diffusion models, trained on billions of image-text pairs, can generate highly realistic visuals from text prompts. However, they are now facing lawsuits from artists alleging the AI replicates their copyrighted works during the training process. Ambient Diffusion, originally presented at the NeurIPS conference in 2023 and recently extended, offers a potential solution.
"Our framework allows for controlling the trade-off between memorization and performance," explained Giannis Daras, a UT Austin computer science graduate student who led the research. "As the level of corruption encountered during training increases, the memorization of the training set decreases."
By training diffusion models through access only to corrupted image data, with up to 90% of individual pixels randomly masked, the Ambient Diffusion approach enables high-quality sample generation without ever exposing the AI to recognizable original source images.
In an experiment using 3,000 celebrity images, a standard diffusion model trained on clean data blatantly copied the training examples when generating new samples. However, when retrained using Ambient Diffusion's corrupted data, the generated faces remained high-fidelity but looked distinctly different from those in the training set.
"The framework could prove useful for scientific and medical applications, too," said Adam Klivans, a UT Austin computer science professor involved in the work. "That would be true for basically any research where it is expensive or impossible to have a full set of uncorrupted data, from black hole imaging to certain types of MRI scans."
The research team, including members from UC Berkeley and MIT, collaborated under the multi-institution Institute for Foundations of Machine Learning directed by Klivans and Alex Dimakis, a UT Austin electrical and computer engineering professor.
Dimakis noted that while some performance trade-off may occur, Ambient Diffusion "points to a solution that will never output noise." This addresses a key challenge as increasingly powerful AI models risk inadvertently infringing copyrights or hallucinating nonexistent data.
The framework's follow-up paper, "Consistent Diffusion Meets Tweedie," recently accepted to the 2024 International Conference on Machine Learning, further extends the approach to train on images corrupted by different types of noise beyond masked pixels, as well as larger datasets.
As debates around AI ethics and intellectual property rage on, the UT Austin team's work represents a significant stride toward developing safe, responsible AI systems capable of high performance without compromising principles.
"Our framework offers an example of how academic researchers are advancing artificial intelligence to meet societal needs," Klivans stated, highlighting the UT Austin's designation of 2024 as the "Year of AI" and focus on impactful AI research.
With Ambient Diffusion, the path toward ethical, copyright-respecting AI generation appears brighter, pointing the way for technology companies and researchers alike to embrace corrupted data as a viable training avenue. As AI capabilities explosively grow, such innovations could steer the field toward greater transparency and responsibility.
Share with friends:
Write and read comments can only authorized users
Last news