2024-05-30
WANDR: a model to generate realistic human motions for avatars
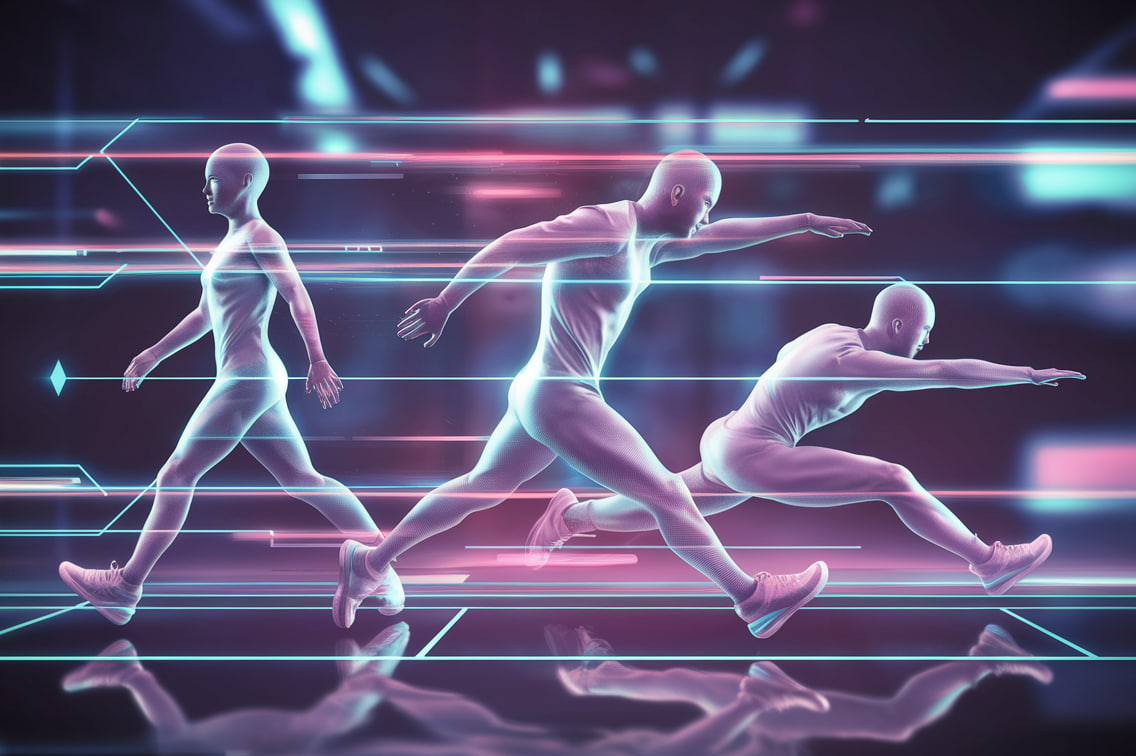
In a groundbreaking development, researchers at the Max Planck Institute for Intelligent Systems and ETH Zurich have unveiled WANDR, a cutting-edge model capable of generating natural human motions for virtual avatars and 3D animated human-like characters. Introduced in a paper to be presented at the upcoming Conference on Computer Vision and Pattern Recognition (CVPR 2024) in June, WANDR unifies different data sources under a single model, paving the way for more realistic motions in the digital realm.
As Markos Diomataris, the first author of the paper, explains, "At a high-level, our research aims at figuring out what it takes to create virtual humans able to behave like us. This essentially means learning to reason about the world, how to move in it, setting goals and trying to achieve them."
The primary objective of this study was to create a model that could generate realistic motions for 3D avatars, allowing them to interact with their virtual environment seamlessly, such as reaching to grab objects. Diomataris emphasizes the complexity of human movements, stating, "Consider reaching for a coffee cup—it can be as straightforward as an arm extension or can involve the coordinated action of our entire body. Actions like bending down, extending our arm, and walking must come together to achieve the goal. At a granular level, we continuously make subtle adjustments to maintain balance and stay on course towards our objective."
Traditionally, there have been two main approaches to teaching virtual agents new skills: reinforcement learning (RL) and compiling datasets of human demonstrations to train machine learning models. While RL allows agents to learn through trial and error, it can be computationally intensive and require careful reward design to prevent unnatural behaviors. On the other hand, using datasets provides richer information about a skill, but few datasets currently include reaching motions, which the researchers aimed to replicate.
Prioritizing motion realism, the team chose to learn this skill from data. "WANDR is the first human motion generation model that is driven by an active feedback loop learned purely from data, without any extra steps of reinforcement learning (RL)," Diomataris explains.
WANDR generates motion autoregressively, frame-by-frame, predicting actions that will progress the avatar to its next state. These predictions are conditioned by time and goal-dependent features, termed "intention," which are recomputed at every frame, acting as a feedback loop to guide the avatar in reaching a given goal using its wrist. This allows WANDR to approach and reach moving or sequential goals, even though it has never been explicitly trained for such tasks.
Existing datasets containing goal-oriented reaching human motions are scarce and do not generalize well across different tasks. To overcome this limitation, the researchers employed a novel approach inspired by behavioral cloning in robotics. During training, a randomly chosen future position of the avatar's hand is considered the goal, allowing WANDR to combine smaller datasets with goal annotations, like CIRCLE, as well as large-scale datasets like AMASS, which lack goal labels but are essential for learning general navigational skills.
By appropriately mixing data from different sources, WANDR produces more natural motions, enabling an avatar to reach arbitrary goals in its environment. "WANDR demonstrates a way to learn adaptive avatar behaviors from data. The 'online adaptation' part is necessary for any real-time application where avatars interact with humans and the real world, like for example, in a virtual reality video game or in human-avatar interaction," Diomataris emphasizes.
The potential applications of WANDR are vast, ranging from generating new content for video games, VR applications, and animated films to enabling more realistic body movements for human-like characters in digital entertainment, AI interfaces, and robotics.
Looking ahead, the researchers plan to explore ways for avatars to leverage large, uncurated datasets of videos to learn movements and interactions with their virtual world, as well as the ability to explore and learn from their own experiences – two fundamental means by which humans acquire experience.
As datasets of human motions continue to grow, the performance of WANDR is expected to improve, bringing us one step closer to truly lifelike virtual avatars capable of mimicking the fluidity and complexity of human movements.
Share with friends:
Write and read comments can only authorized users
Last news