2024-04-04
A new model of Explicable Artificial Intelligence (XAI)
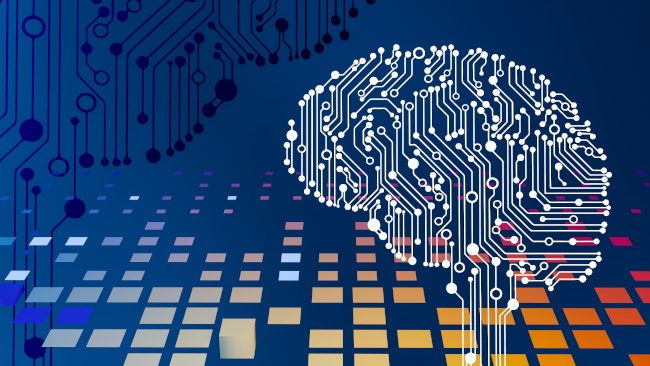
University of Waterloo researchers have developed an innovative Explainable AI (XAI) model that reduces bias and improves confidence in machine learning-driven healthcare decisions. In medicine, biased algorithms can have serious consequences, causing certain groups to be overlooked or misdiagnosed. The new approach aims to unravel complex data patterns to reveal underlying causes unaffected by anomalies or incorrect labels.
In hospitals, staff rely on datasets and algorithms to guide critical patient care choices. But machine learning can propagate biases if minority symptoms go unnoticed or data mislabeling distorts results. Such issues lead to unfair, inaccurate diagnoses.
Led by Dr. Andrew Wong, the new study analyzes extensive protein binding data from X-ray crystallography. By revealing hidden statistics of amino acid interactions obscured at the data level, the team shows how confusing statistics can be disentangled to yield missed insights.
This discovery led to developing the Pattern Discovery and Disentanglement (PDD) XAI system. As lead researcher Dr. Peiyuan Zhou explained, PDD bridges the gap between AI and human understanding for robust decisions and knowledge discovery.
Co-author Professor Annie Lee sees tremendous value for PDD in clinical settings. Case studies demonstrate PDD can predict outcomes from medical histories and detect rare patterns to compare against similar anomalies. This allows flagging incorrect machine learning labels for more accurate individual diagnoses.
"PDD represents a significant XAI contribution," said Wong. "We've shown for the first time that entangled statistics can be unraveled to reveal deep knowledge missed at the data level."
The results enable professionals to make reliable diagnoses backed by statistical explanations, leading to better treatment recommendations. PDD also tracks atypical cases to add them to the database, increasing diagnostic precision over time.
By elucidating hidden relationships in medical data, the researchers' innovative XAI approach reduces biases and builds trust in AI-assisted healthcare. PDD exemplifies how AI transparency and human understanding can be combined for more accurate, ethical decision making. Such hybrid systems will become increasingly critical as machine learning permeates high-stakes domains like medicine.
Share with friends:
Write and read comments can only authorized users
Last news